By Beth Barrow, Conservation Research Director
Our team just spent over a year in the field carrying out an orangutan population survey inside Gunung Palung National Park. This wasn’t just your average population survey though; this one was designed to compare traditional methods with a new method using drone technology. Traditionally, observers walk line transects in search of orangutan nests, recording data for each nest they come across, which is later used to calculate an estimated density of orangutans in a given area. It’s a time consuming and costly method. Observers are usually able to cover only around 2km of transect each day. To put that into context, our total walked transect length for this recent survey was just shy of 100km – no mean feat! Each month, our team spent around 20 days in the field, surveying areas using this traditional ground survey method, after which we enlisted the help of Aerozone, a drone consultancy company based in Jakarta, to fly drones over the transect area we had just surveyed by ground. We designed the survey to encompass both primary and disturbed rainforest, from around 0 to 1120 meters above sea level, across eight different habitat types. This design allows us to compare differences in nest detection in drone imagery throughout several distinct orangutan habitat areas within the National Park.
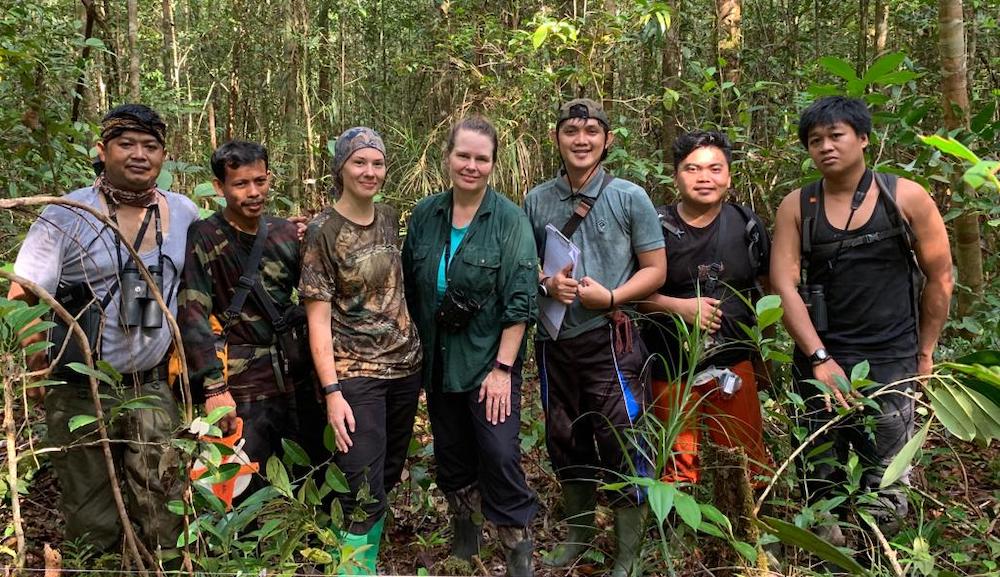
Although the field work has now come to an end, there is much work still to be done. Our drone surveys collected around 10,000 images in total, and each individual image must be scanned manually in search of orangutan nests. The first few months of analysis time were spent devising a working method for this process, with a lot of time spent testing out different methods and ironing out kinks in our approach. After settling on a method that allows us to accurately and efficiently search for and tag nests in the imagery, and then further compare our results with traditional nest counts in GIS software, we put together a team of 6 people to work on the analysis remotely, in the UK and US. Using Gnu Image Manipulation Program, we overlay a grid on a drone image and proceed to scroll through, searching grid-by-grid, for orangutan nests. Each time the observer finds a nest, they circle it in red and save a copy of the image. The drone images have an overlap of around 75%, meaning that a single nest is likely to be visible in up to 6 images. An important aspect of our method involves ensuring every duplicate nest is circled in every image, otherwise the next step of the analysis won’t work. A single transect is made up of on average of 150 images and, depending on nest density, can take anywhere from 2-4 days to complete. With a total of 50 transects to analyse, that’s 100-200 days of work dedicated to the big nest search. As you might be pondering as you read this, this aspect of the work is nowhere near as fun as searching for nests on the ground in the Indonesian rainforest!
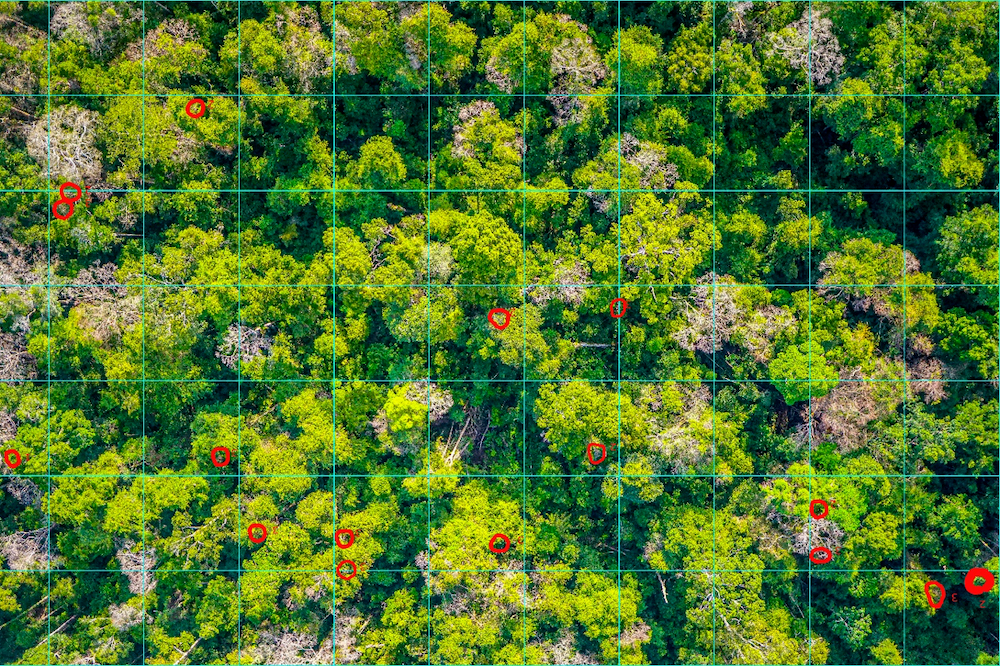
Once all images for a transect have been searched and any nests tagged, I then use those edited images to build an orthomosaic in Agisoft Metashape Professional. This program stitches together all the drone imagery, removing any overlap, and therefore removing any duplicate nests. The resulting mosaic is one rectangular image showing the forest canopy, with GPS information embedded, covering an area of around 1km by 100m, complete with red circles indicating unique nest locations. This image is then imported into GIS software where I overlay the GPS coordinates for each nest we found during our ground survey of that transect. Zooming in, I am able to see an estimated number of potentially ‘matched’ nests – those found both by drone and by ground survey – as well as the nests that were only found using one of the survey methods. To take this one step further, I then go to each GPS point for a nest we found on the ground but that did not seem to have been found by drone. I pinpoint the location, open the original image and search that area to see if a nest is visible but not initially detected or positively identified as a nest. This extra step allows us to get a rough estimate for observer error when manually searching images for orangutan nests. Eventually, once the images for all 50 transects have been searched and results analysed using GIS software, we will be able to use our final nest counts, along with data collected from the ground survey, to formulate a new calculation, converting drone nest counts to orangutan population density and estimated population size.
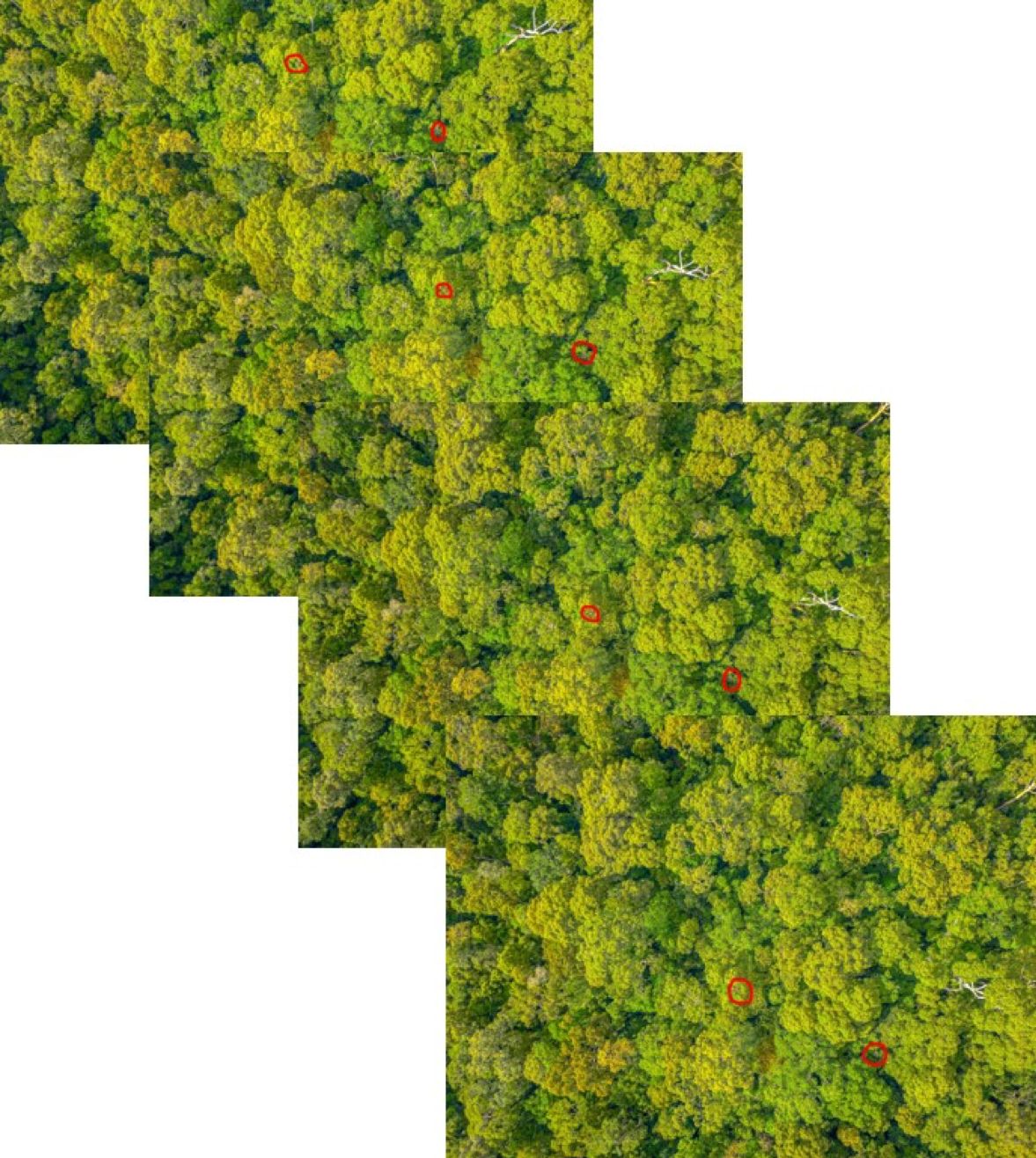
Searching for nests in these images might not sound like a difficult task, but it can very much like looking for a needle in a haystack. Observers have to confidently differentiate an orangutan nest from broken tree branches, or epiphytic plants (small leafy plants that grow on the surface of a tree), or just simply from discoloured, dead leaves within the canopy. From a bird’s eye view, from far away, this is not as easy as it sounds! We are now building a database of nests that we have confirmed with certainty through comparing GPS points with nests we saw close up on the ground which will allow easier training for nest identification in the future.
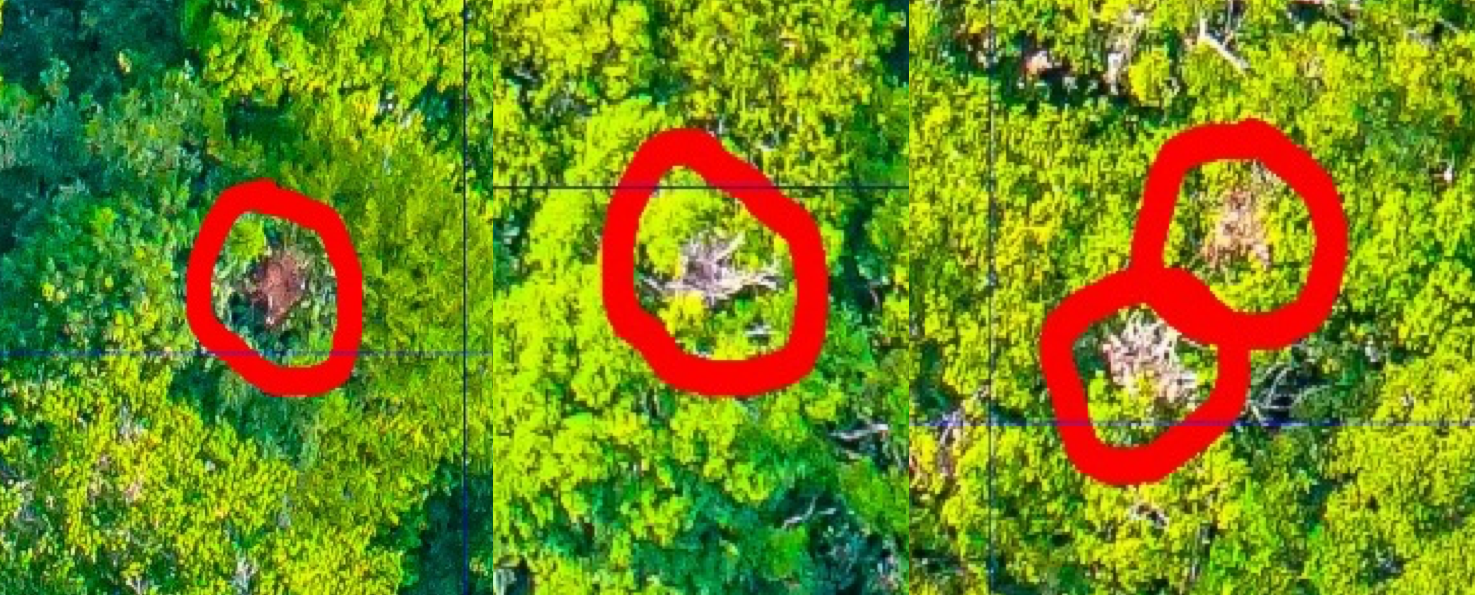
Additionally, and perhaps most excitingly for the future application of this potential method, we are currently using our dataset to assist conservation drone expert, Dr. Serge Wich, from the University of Liverpool, UK, in his work on an automated technique for nest detection in drone imagery through artificial intelligence software. Our tagged nests will be used as part of this larger project to build a database aimed at training this AI program to automatically detect nests in drone imagery. The success of this software would be instrumental in the practical application of using drones to survey orangutan populations, reducing the time spent analysing imagery and allowing surveys to take place more frequently so that populations of this critically endangered ape can be better monitored through time.
So, although the time investment in this project is long and the work ever so slightly tedious, our team are excited to see the results and are already hopeful that this method will indeed be a realistic tool for the long-term monitoring of orangutans across their entire range.
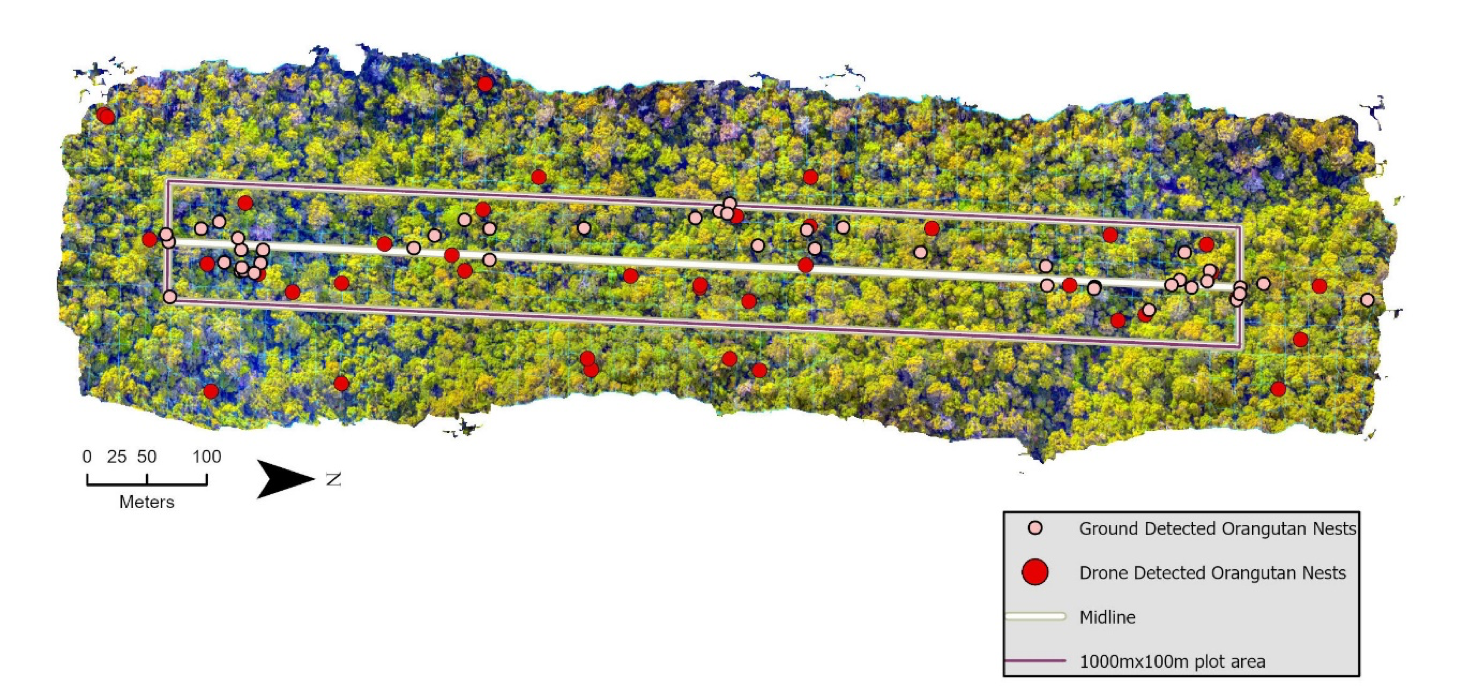